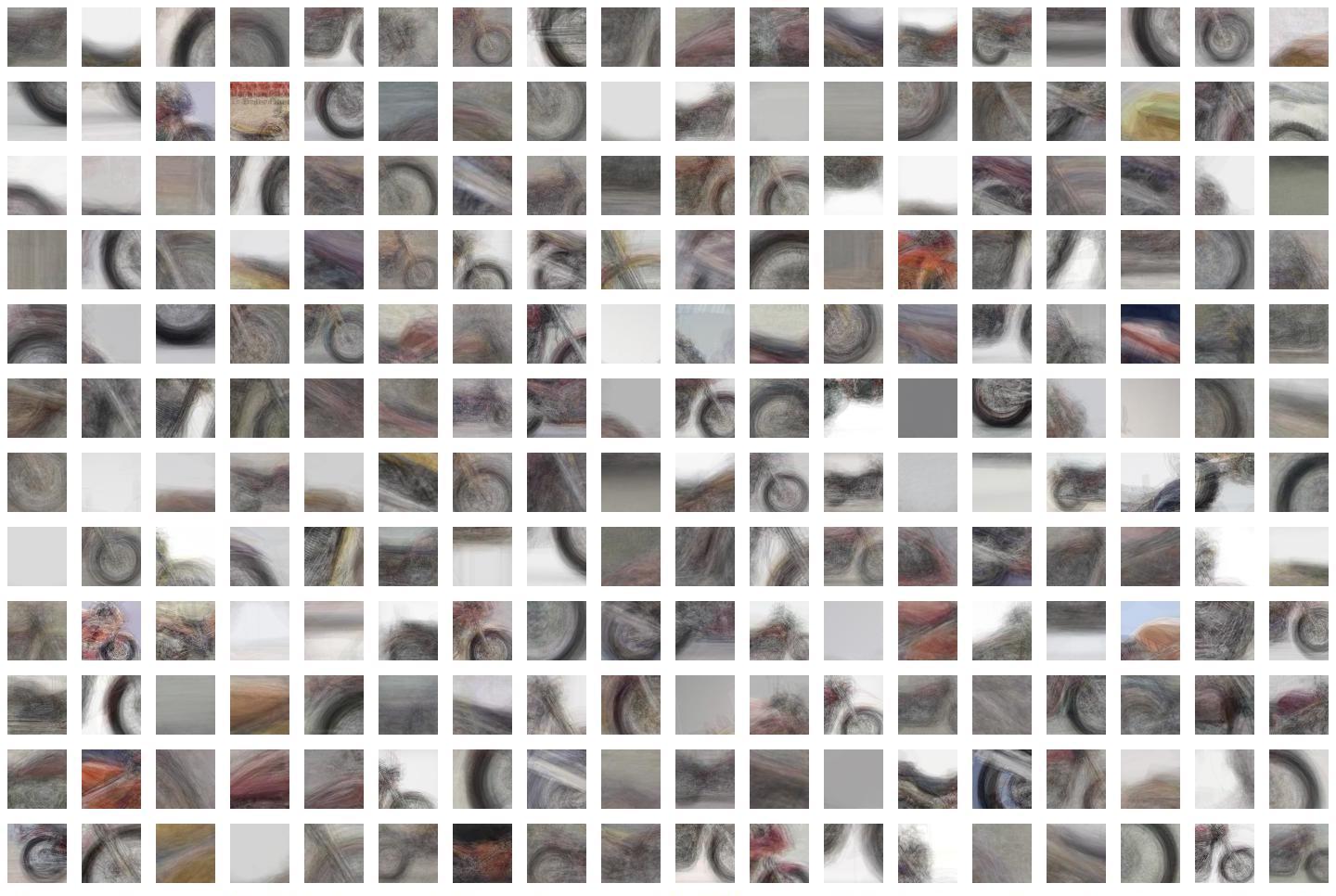
IMAGE: A ‘computer vision’ system developed at UCLA can identify objects based on only partial glimpses, like by using these photo snippets of a motorcycle. view more
Credit: UCLA Samueli
Researchers from UCLA Samueli School of Engineering and Stanford have demonstrated a computer system that can discover and identify the real-world objects it “sees” based on the same method of visual learning that humans use.
The system is an advance in a type of technology called “computer vision,” which enables computers to read and identify visual images. It is an important step toward general artificial intelligence systems–computers that learn on their own, are intuitive, make decisions based on reasoning and interact with humans in a more human-like way. Although current AI computer vision systems are increasingly powerful and capable, they are task-specific, meaning their ability to identify what they see is limited by how much they have been trained and programmed by humans.
Even today’s best computer vision systems cannot create a full picture of an object after seeing only certain parts of it–and the systems can be fooled by viewing the object in an unfamiliar setting. Engineers are aiming to make computer systems with those abilities–just like humans can understand that they are looking at a dog, even if the animal is hiding behind a chair and only the paws and tail are visible. Humans, of course, can also easily intuit where the dog’s head and the rest of its body are, but that ability still eludes most artificial intelligence systems.
Current computer vision systems are not designed to learn on their own. They must be trained on exactly what to learn, usually by reviewing thousands of images in which the objects they are trying to identify are labeled for them.
Computers, of course, also cannot explain their rationale for determining what the object in a photo represents: AI-based systems do not build an internal picture or a common-sense model of learned objects the way humans do.
The engineers’ new method, described in the Proceedings of the National Academy of Sciences, shows a way around these shortcomings.
The approach is made up of three broad steps. First, the system breaks up an image into small chunks, which the researchers call “viewlets.” Second, the computer learns how these viewlets fit together to form the object in question. And finally, it looks at what other objects are in the surrounding area, and whether or not information about those objects is relevant to describing and identifying the primary object.
To help the new system “learn” more like humans, the engineers decided to immerse it in an internet replica of the environment humans live in.
“Fortunately, the internet provides two things that help a brain-inspired computer vision system learn the same way humans do,” said Vwani Roychowdhury, a UCLA professor of electrical and computer engineering and the study’s principal investigator. “One is a wealth of images and videos that depict the same types of objects. The second is that these objects are shown from many perspectives–obscured, bird’s eye, up-close–and they are placed in different kinds of environments.”
To develop the framework, the researchers drew insights from cognitive psychology and neuroscience.
“Starting as infants, we learn what something is because we see many examples of it, in many contexts,” Roychowdhury said. “That contextual learning is a key feature of our brains, and it helps us build robust models of objects that are part of an integrated worldview where everything is functionally connected.”
The researchers tested the system with about 9,000 images, each showing people and other objects. The platform was able to build a detailed model of the human body without external guidance and without the images being labeled.
The engineers ran similar tests using images of motorcycles, cars and airplanes. In all cases, their system performed better or at least as well as traditional computer vision systems that have been developed with many years of training.
###
The study’s co-senior author is Thomas Kailath, a professor emeritus of electrical engineering at Stanford who was Roychowdhury’s doctoral advisor in the 1980s. Other authors are former UCLA doctoral students Lichao Chen (now a research engineer at Google) and Sudhir Singh (who founded a company that builds robotic teaching companions for children).
Singh, Roychowdhury and Kailath previously worked together to develop one of the first automated visual search engines for fashion, the now-shuttered StileEye, which gave rise to some of the basic ideas behind the new research.
Disclaimer: AAAS and EurekAlert! are not responsible for the accuracy of news releases posted to EurekAlert! by contributing institutions or for the use of any information through the EurekAlert system.