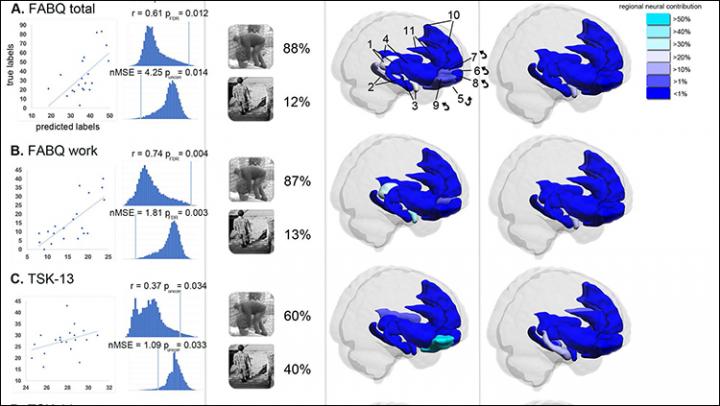
IMAGE: The model performance (r, MSE) characterizes the strength of relationship between true and predicted labels. Condition and region weights show the predictive contribution of the two different conditions (harmful, harmless)
Credit: Meier et al., eNeuro (2018)
Researchers applied a machine learning technique that could potentially translate patterns of activity in fear-processing brain regions into scores on questionnaires used to assess a patient’s fear of pain. This neuroscientific approach, reported in eNeuro, may help reconcile self-reported emotions and their neural underpinnings.
Pain-related fear is typically assessed with various questionnaires, often used interchangeably, that ask patients how they feel about their clinical pain. However, it is unclear to what extent these self-reports measure fear and anxiety, which are known to involve different brain regions, and perhaps other psychological constructs.
Michael Meier and colleagues from Petra Schweinhardts’ lab at the Balgrist University Hospital in Zurich, Switzerland, addressed this ambiguity by imaging the brains of patients with low back pain as they watched video clips evoking harmful (bending) and harmless (walking) activities for the back. Participants’ brain activity was predictive of their scores on the various questionnaires. Importantly, different questionnaires were associated with distinct patterns of neural activity. These results suggest similar questionnaires may measure different emotional states.