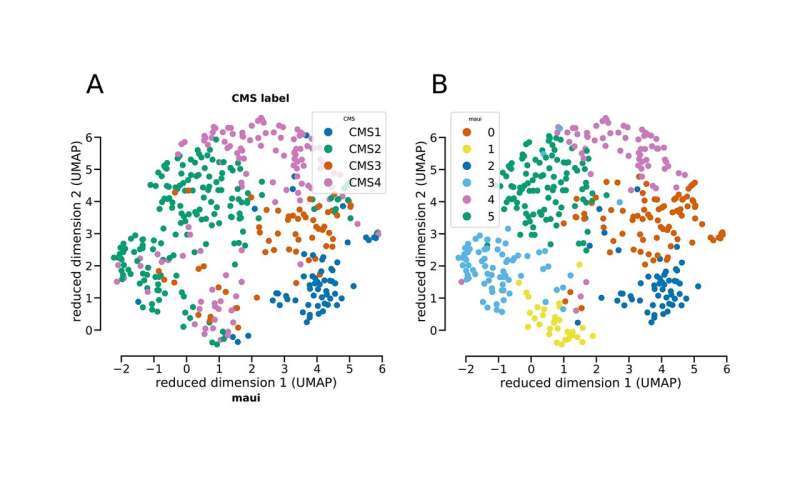
A new deep-learning algorithm can quickly and accurately analyze several types of genomic data from colorectal tumors for more accurate classification, which could help improve diagnosis and related treatment options, according to new research published in the journal Life Science Alliance.
“Data science can handle complex data that is hard to handle other ways and makes sense of it,” Akalin said. “You can feed it everything you have on the tumors and it finds meaningful patterns. “Deep learning identifies molecular patterns of cancerRonen and Akalin discuss the research results.
The program was not just more accurate, it also works much faster than other machine-learning algorithms—three minutes to pick out 100 patterns compared to the other programs, which took 20 minutes and 11 hours. “It is able to learn orders of magnitude more latent factors, at a fraction of the computation time,” said Jonathan Ronen, first author of the research.
The team was surprised at how fast the system performs, especially because they did not have to use GPUs to speed up calculations. This shows how extremely well optimized the algorithm is, though they are continuing to fine-tune the system.
Improving drug discovery.
The team, which also included Bayer AG computational biologist Sikander Hayat, adapted their program to analyze cell lines taken from tumors and grown in labs for researching the effects of potential drug treatments. However, cell lines differ on the molecular level from real tumors in many ways.
The team used MAUI to compare cell lines currently used for testing colorectal cancer drugs to see how closely they were related to real tumors. Nearly half of the lines were found to be more related to other cell lines than actual tumors. A handful were found to be the best lines most closely representing the different classes of CRC tumors.While drug discovery research is moving away from cell lines, this insight could help maximize the potential impact of cell line research, and could be adapted for other types of genetic-based drug testing tools.Google for tumors.
Now that the deep-learning platform for colorectal cancer has been established, it could be used to analyze data for new patients. “Think of this like a search engine,” Akalin said. A clinician could input the new patient’s genetic data into MAUI to find the closest match to quickly and accurately classify the tumor.
The platform could advise what drugs have been used on the closest matching tumors and how well they worked, thus helping to predict drug responses and survival outlook. For now, this could take place in a research setting only after doctors have tried the established protocols. It is a long road for a test or system to be approved for clinical use, Akalin said.
The team is exploring the potential for commercialization with the help of the Berlin Institute of Health’s Digital Health Accelerator Program. They are also in the process of adapting MAUI for other types of cancers.
Max Delbrück Center for Molecular Medicine