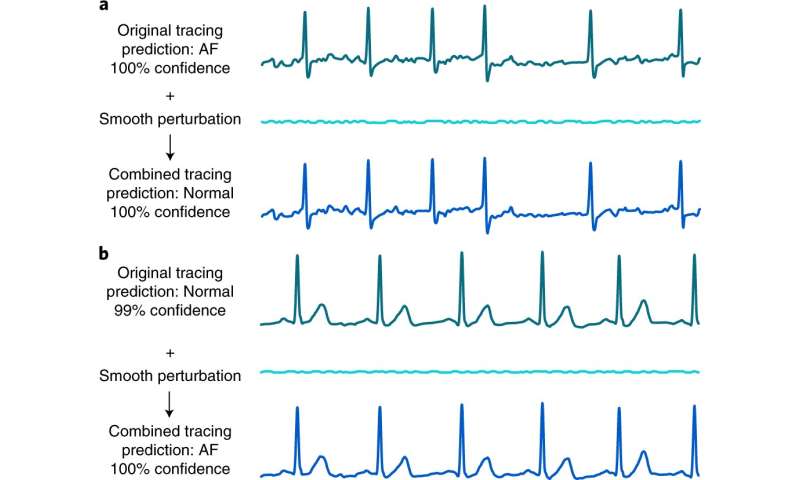
A team of researchers from New York University and NYU Langone Health has found that deep learning electrocardiogram devices can be susceptible to adversarial attacks. In their paper published in the journal Nature Medicine, the group describes how they developed an attack approach and tested it with electrocardiogram devices.
Over the past several years, deep-learning systems have been found to be very good at certain tasks that are challenging for humans—playing chess, for example, or finding faces in a crowd. Deep-learning systems have also been applied to medicine—to help detect breast cancer tumors, for example, or to monitor vital signs such as the heartbeat to alert doctors or patients to problems. One such application is the electrocardiogram acquisition system, in which a device monitors the heart and analyses an electrocardiogram—a record of a person’s heartbeat. Deep learning algorithms have been developed and trained to look for abnormalities that might indicate such activity as irregular atrial fibrillation. And devices such as smartwatches have been designed to use them. In this new effort, the researchers have found evidence that such devices and the output they produce can be susceptible to an attack.
The researchers demonstrated a vulnerability in deep-learning electrocardiogram devices by first obtaining a set of thousands of ECG recordings, which they separated into one of four groups: those that were normal, those that showed atrial fibrillation, other or noise. After splitting up the data and training their system on their own convolutional network they introduced a small bit of noise to samples in a test set—too small for humans to make out, but just enough to make the AI system think that it was seeing atrial fibrillation. And testing showed it worked as planned. The system wrongly identified normal electrocardiograms as examples of atrial fibrillation in 74 percent of the graphs tested. The researchers also showed the adversarial examples to two clinicians—they were not as easily fooled. They only found that 1.4 percent of the readings were mislabeled.
The researchers acknowledge that it would be difficult for a hacker to replicate their efforts with data from a real electrocardiogram device such as a smartphone, because it would require direct access—something that is not likely to be possible in the real world. But still, they suggest that their efforts indicate that AI-based medical devices should be tested for vulnerabilities before being approved for use by the public.
Medical Xpress