
Patient data are a treasure trove for AI researchers. There’s a problem though: many algorithms used to mine patient data act as black boxes, which makes their predictions often hard to interpret for doctors. Researchers from Eindhoven University of Technology (TU/e) and the Zhejiang university in China have now developed an algorithm that not only predicts hospital readmissions of heart failure patients, but also tells you why these occur. The work has been published in BMC Medical Informatics and Decision Making.
Doctors are increasingly using data from electronic healthcare records to asses patient risks, predict outcomes, and recommend and evaluate treatments. Application of machine learning algorithms in clinical settings has however been hampered by lack of interpretability. The models often act as black boxes: you see what goes in (data) and what comes out (predictions), but you can’t see what happens in between. It can therefore be very hard to interpret why the models are saying what they are saying.
This undermines the trust healthcare professionals have in machine learning algorithms, and limits their use in everyday clinical decisions. Of course, interpretability is also a key requirement of EU privacy regulations (GDPR), so improving it also has legal benefits.
Attention-based neural networks
To solve this problem, Ph.D. candidate Peipei Chen of the Department of Industrial Engineering and Innovation Sciences, together with other researchers at TU/e and Zhejiang University in Hangzhou, has tested an attention-based neural network on heart patients in China. Attention-based networks are able to focus on key details in data using contextual information.
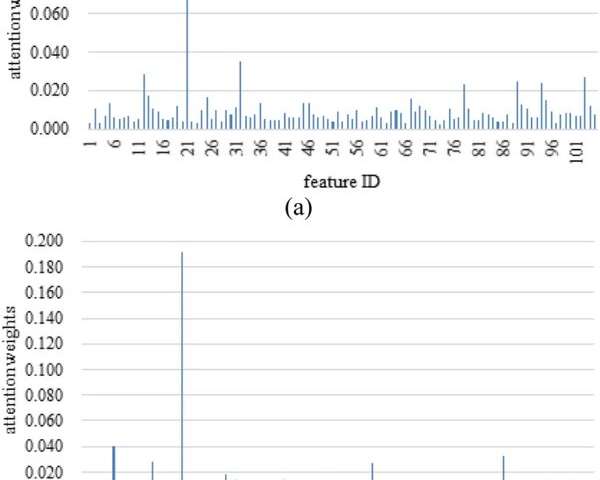
This is the same approach humans take to evaluate the world around them. When people look at a picture of a Dalmatian, they immediately focus on the four-legged black-spotted white shape in the center of the image and recognize it’s a dog. To do this, they apply both intuition and information gleaned from the context. Attention-based neural networks essentially do the same.
Because of their sensitivity to context, these neural networks are not only good at making predictions, they also allow you to exactly see what feature was responsible for what result. Of course, this considerably increases the interpretability of your predictions. Attention-based networks are traditionally used in image recognition and speech recognition, where context is key in understanding what’s going on. Recently, they have also been applied in other domains.
Experiment
Peipei Chen and her colleagues followed 736 heart failure patients from a Chinese hospital. Based on patient characteristics, they tried to predict and interpret readmissions of the patients within 12 months after their release from hospital. The researchers looked at 105 features, including age and gender, blood pressure and heart rate, diseases such as diabetes and kidney problems, length of stay and medicine use.
The attention-based model predicted two-thirds of all readmissions, slightly improving on three other popular prediction models. More importantly, the model was able to specify what risk factors were contributing most to chance of readmission for each patient (see image), making the predictions much more useful for doctors. Moreover, the model provided the most important risk factors for all patient samples. Doing so, the researchers identified three echocardiographic measurements that were not identified by another model.
Before the attention-based model can be implemented by doctors, it needs to be validated on larger data sets. Chen also wants to expand on the research by including textdata gained from discharge and daily progress notes in the electronic health records.
Henk Van Appeven, Eindhoven University of Technology