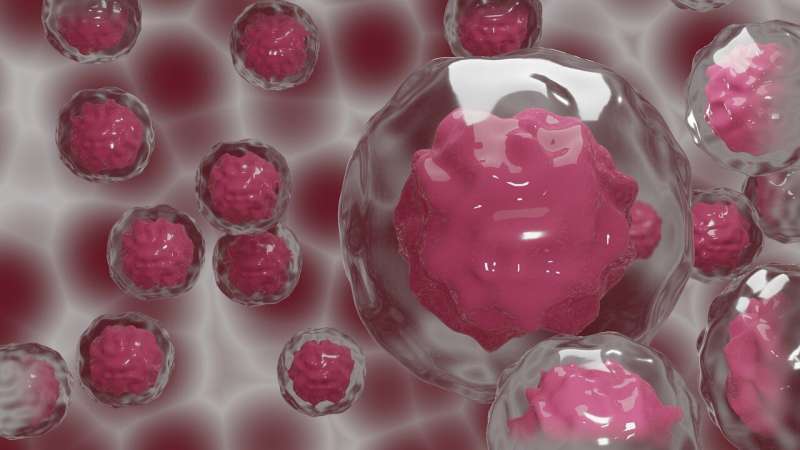
Researchers at the Cheriton School of Computer Science have applied machine learning to identify tumor-specific antigens, which could help make personalized cancer vaccines practically feasible and more accurate.
In cancer, when a mutation occurs in a cell’s DNA, a substitution takes place. This substitution is flagged as an invader by our immune system and is referred to as a neoantigen, a mutated peptide that appears on the surface of cancer cells.
“If we can figure out what the neoantigens are on cancer cells, they can be used to develop a cancer vaccine—a vaccine that’s personalized to the cancer patient and which uses the patient’s own immune system to attack the tumor,” explains Hieu Tran, adjunct professor at the Cheriton School of Computer Science.
“When a cell becomes cancerous, the body knows about it,” adds Ming Li, a University professor at the Cheriton School of Computer Science, who also holds the Canada Research Chair in Bioinformatics. “That’s because the human leukocyte antigen or HLA system—which is responsible for the regulation of the immune system—can showcase whether a peptide on the cell’s surface is normal or mutated. If the HLA system presents a normal peptide, our immune system doesn’t attack it. Our immune system will attack only the cells with mutations, the ones with neoantigens, otherwise known as cancerous tumor cells, on their surface.”
The trick, however, is finding these tumor-specific neoantigens—essentially a needle in a large haystack. Not surprisingly, it is a bewilderingly difficult task to do using conventional methods, but it is crucially important when developing a personalized cancer vaccine.
Catering medicine to the individual
Amino acids are the building blocks of peptides and ultimately protein molecules. Without them, we wouldn’t have an immune system, be able to digest food, grow or be able to procreate. By convention, amino acids are labeled using a one-letter code. For example, the amino acid alanine is labeled A, arginine is labeled R, asparagine is labeled N, and so on. A peptide’s amino acid sequence can be considered as a word composed of these letters.
“If you are familiar with natural language processing, you’ve likely seen your mobile phone guess the next word you might have typed as you compose a message. You write ‘how’ and it suggests ‘are’ and if you type ‘are’ it suggests ‘you,'” Hieu Tran said.
“We applied a similar machine-learning model to determine the amino acid sequence of neoantigens based on this one-letter amino acid code. If I know your immunopeptidome—the thousands of short eight to 12 amino acid peptide antigens displayed on the cell surface—and I know that a neoantigen is different from your existing peptides by just one mutation, I can train a machine learning model using your normal peptides to predict the mutated peptides. We used a recurrent neural network—a machine learning model we call DeepNovo—to predict the amino acid sequence of neoantigens.”
To do this, the researchers downloaded the immunopeptidome datasets of five patients with melanoma, a type of skin cancer, which they then used to train, validate and test their machine learning model.
Even more impressively, the machine learning model is able to personalize the results—that is, it identifies specific neoantigens for each individual patient to provide personalized treatment and care.
“Cancer immunotherapy is quickly becoming a fourth modality of cancer treatment, alongside surgery, chemotherapy and radiotherapy,” adds Ming Li. “Every patient is different and every cancer is different, so cancer treatment shouldn’t be the same for all. Treatment should be tailored to the patient and that’s what our personalized machine learning model allows us to do.”
Joe Petrik, University of Waterloo