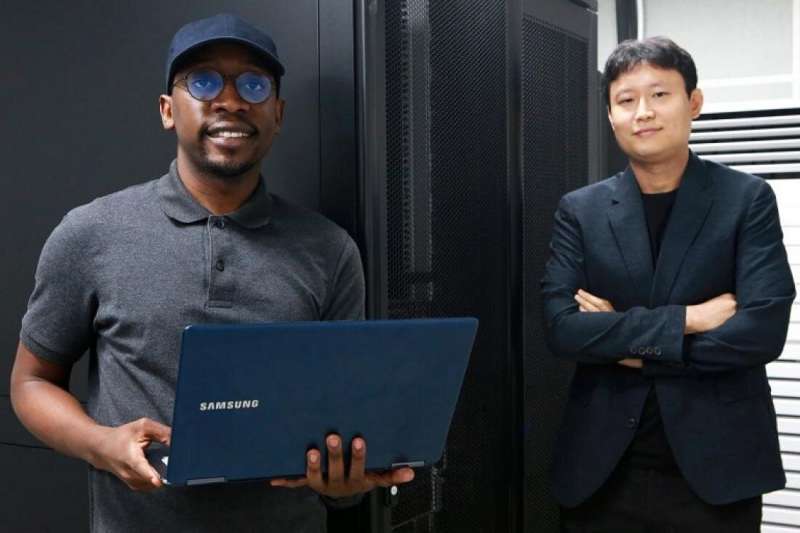
The current gold standard for COVID-19 diagnosis is a nasal swab followed by reverse transcription polymerase chain reaction. But such tests are time consuming, requiring days before results are available. This wastes crucial time in the treatment and prevention of the disease. Recently, scientists from Korea have developed a computerized framework that can swiftly and accurately interpret chest CT scans to provide COVID-19 diagnosis in minutes, potentially changing how we tackle this disease.
In a little over 18 months, the novel coronavirus (SARS-CoV-2) has infected over 18 million people and caused more than 690,000 deaths. The current standard for diagnosis through reverse transcription polymerase chain reaction is limited owing to its low sensitivity, high rate of false positives, and long testing times. This makes it difficult to identify infected patients quickly and provide them with treatment. Furthermore, there is a risk that patients will still spread the disease while waiting for the results of their diagnostic test.
Chest CT scans have emerged as a quick and effective way to diagnose the disease, but they require radiologist expertise to interpret, and sometimes the scans look similar to other kinds of lung infections, like bacterial pneumonia. Now, a new paper in Medical Image Analysis by a team of scientists, including those from Daegu Gyeongbuk Institute of Science (DGIST), South Korea, details a technique for the automated and accurate interpretation of chest CT scans. “As academics who were equally affected by the COVID pandemic, we were keen to use our expertise in medical image analysis to aid in faster diagnosis and improve clinical workflows,” says Prof. Sang Hyun Park and Philip Chikontwe from DGIST, who led the study.
To build their diagnostic framework, the research team used a Machine Learning technique called “Multiple Instance Learning” (MIL). In MIL, the machine learning algorithm is “trained” using sets, or “bags,” of multiple examples called “instances.” The MIL algorithm then uses these bags to learn to label individual examples or inputs. The research team trained their new framework, called dual attention contrastive based MIL (DA-CMIL), to differentiate between COVID and bacterial pneumonia, and found that its performance was on par to other state-of-the-art automated image analysis methods. Moreover, the DA-CMIL algorithm can leverage limited or incomplete information to efficiently train its AI system.
“Our study can be viewed from both a technical and clinical perspective. First, the algorithms introduced here can be extended to similar settings with other types of medical images. Second, the ‘dual attention,’ particularly the ‘spatial attention,’ used in the model improves the interpretability of the algorithm, which will help clinicians understand how automated solutions make decisions,” explain Prof. Park and Mr. Chikontwe.
This research extends far beyond the COVID pandemic, laying the foundation for the development of more robust and cheap diagnostic systems, which will be of particular benefit to under-developed countries or countries with otherwise limited medical and human resources.
Daegu Gyeongbuk Institute of Science and Technology