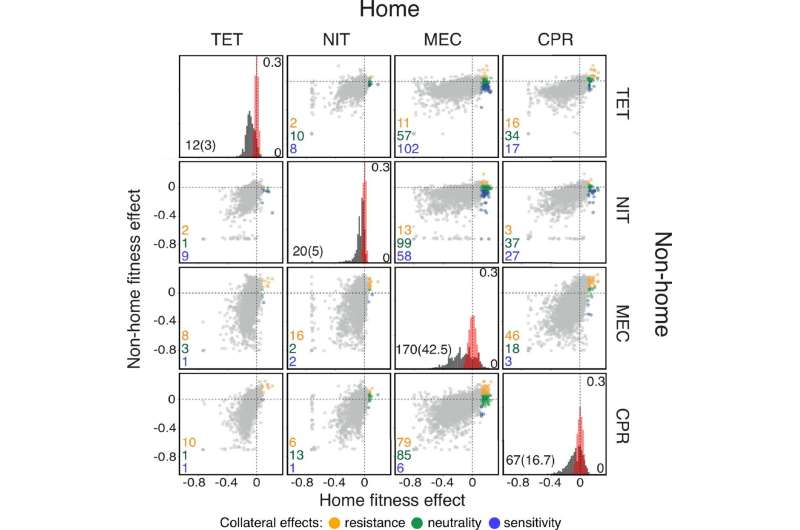
Bacteria have dangerously evolved to thwart many of the medicines that were designed to kill them. As a result, a growing antibiotic resistance crisis is responsible for more than 700,000 deaths each year, emerging as one of the world’s most pressing health issues.
Since the development of new antibiotics to treat infections has stalled, many patients now receive treatments based on multiple drugs in the hopes that their joint therapeutic effects can forestall the evolution of further resistance. Yet there are plenty of risks and unknowns involved in such multi-drug treatments.
Giving one drug to a patient often causes bacteria to evolve resistance against it. Fortunately, some of these resistant mutants become more susceptible to a second drug, which allows doctors to successfully treat the infection. However, doctors can’t always be sure when and if evolution will take this fortunate course. Even worse, resistance against the initial drug can backfire and cause an increase in resistance against the second drug, leaving the doctors without any further treatment options.
University of California San Diego scientists have now developed a way that can help doctors calculate the odds of the fortuitous outcomes for different drug pairs and thereby boost the odds of a successful treatment. As described in the journal eLife, graduate student Sarah Ardell and Assistant Professor Sergey Kryazhimskiy developed a mathematical model that can calculate the risk of resistance evolution for various drug pairs.
“The problem with using multiple drugs to treat bacteria is that we just don’t know which mutations are available to bacteria,” said Kryazhimskiy, of the Division of Biological Sciences’ Section of Ecology, Behavior and Evolution. “In many situations, bacteria can have access to mutations that make them resistant to both drugs as well as to mutations that make them resistant to the first drug but susceptible to the second one. In such situations, it’s very difficult to predict which way the population will evolve. The model we developed allows us to make these predictions.”
In developing the model, Ardell and Kryazhimskiy used a new concept called “JDFE,” which stands for “joint distribution of fitness effects (of new mutations).” JDFE characterizes the various kinds of mutations available to bacteria and allows researchers to classify drug pairs into those that facilitate or hinder multi-drug resistance.
Having looked at mutational data available for the bacterium Escherichia coli, the researchers discovered many resistance mutations against various commonly used antibiotics that lead to collateral sensitivity (a beneficial outcome) or collateral resistance (a detrimental outcome) with other drugs. They say their new model could help better predict resistance outcomes, which signifies a win for infected patients, though it is not foolproof given the unavoidable randomness of evolution.
Ardell said she was surprised to learn that antibiotic resistance cannot be thought of as a simple deterministic process. The more she learned the more it became clear that different bacterial populations evolve resistance in different ways, even in controlled lab conditions. The same experiments carried out by different labs often produce contradictory results, she found.
The strain of bacteria, concentration of drugs and the nutrients in the organism’s environment can all lead to a mixed bag of results.
“But even if all of those things are exactly the same you could still get different outcomes in two different iterations just because evolution builds up by random mutations,” Ardell said. “Two different populations could have randomly accumulated different mutations with different collateral effects, even if everything else is equal. There is so much variability and randomness in these processes, which is an incredibly important thing to think about for patients. We want to give drug pairs that we are confident that will, as much as possible, produce collateral sensitivity—and not just 50 percent of the time.”
The researchers indicate there is still a lot to learn about the diversity of collateral effects of resistance mutations.
Ardell is now studying pairs of drugs that treat the same target, the ribosome, an important protein complex within bacterial cells. She is building a metabolic model of the cell to understand JDFE from a mechanistic perspective.
“The crux of our result is that we can predict the probability of evolving collateral resistance,” said Kryazhimskiy. “It’s not perfect but it’s preferable to having no idea what will happen at all. If we choose the drug pairs carefully, we can minimize the probability of collateral resistance. We can’t completely preclude the adverse outcome, but we can minimize the chance that it will happen. Our work may eventually help clinicians choose drugs that minimize the evolution of multidrug resistance.”
Mario Aguilera, University of California – San Diego