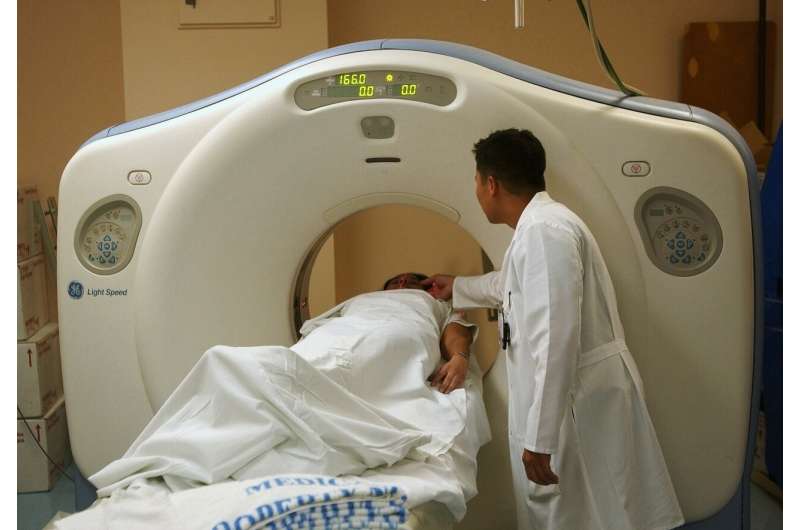
Artificial intelligence is poised to revolutionize the field of radiology as a tool to improve disease detection, diagnosis, and clinical care. The technology has the potential to assist clinicians by uncovering hidden information within imaging scans invisible to even the well-trained eye.
In a paper published in JAMA Oncology, Columbia University researchers demonstrate that applying artificial intelligence to standard-of-care imaging can help predict how well immunotherapy will work for patients with melanoma. In particular, they developed a machine learning algorithm that analyzes a patient’s computed tomography (CT) scans and creates a biomarker—known as a radiomic signature—that correlates with patient outcome.
The signature used specific features of the tumor to determine with high accuracy whether a given individual’s disease would respond well to immunotherapy, remain stable, or continue to progress. The goal of immunotherapy, which has become a primary treatment for melanoma, is to stimulate a patient’s own immune system to fight cancer.
“We hope to take a patient early on who looks like they are not doing well on a given therapy because of their signature and enhance, change, or add another drug to the therapy,” says Lawrence H. Schwartz, MD, the James Picker Professor and chair of the Department of Radiology at Columbia University Vagelos College of Physicians and Surgeons (VP&S). “[The goal is to] really optimize an individualized cancer care for each patient in real time.”
Dr. Schwartz, a member of the Herbert Irving Comprehensive Cancer Center (HICCC), aims to expand the project with his colleagues to a variety of different tumor types—such as lung cancer, colon cancer, renal cancer, and prostate cancer—as well as other treatments beyond immunotherapy. The researchers wanted to start with a novel therapy and chose melanoma because of the recent, rapid adoption of immunotherapy for the disease.
Currently, clinicians rely almost entirely on tumor size to estimate the benefit of a therapy. Patients receive a baseline CT scan and then subsequent follow-up scans after treatment has begun. If the tumor shrinks, the treatment seems to be working, while growth implies that the patient’s disease is getting worse.
But this is not necessarily the case with immunotherapy, and studies have shown that tumor size and growth does not always correlate with overall survival.
“Most of the current response criteria were developed several decades ago to assess the response to systemic treatments like chemotherapy,” says the study’s first author Laurent Dercle, MD, Ph.D., an associate research scientist in the Department of Radiology at VP&S. “Immunotherapy has new patterns of response and progression, with some patients having a transitory increase in tumor size and then a response. Because of that, we needed to create new tools in order to predict treatment success.”
Biologically, tumors may evolve throughout the course of a patient’s disease in ways that are more complex than a measure of size alone can reflect. As an example of this, the researchers found that their machine learning algorithm worked best when it took not only tumor volume and growth into account, but also tumor spatial heterogeneity, or the non-uniform distribution of cancer cells across disease sites, and texture, which looks at the variation of pixel intensities across the tumor CT image.
The researchers validated the algorithm on data from 287 patients with advanced melanoma who participated in the KEYNOTE-002 and KEYNOTE-006 multicenter clinical trials, which administered the immunotherapy drug pembrolizumab. The radiomic signature, which used CT images obtained at baseline and 3-month follow-up, was able to estimate overall survival at 6 months with a high degree of accuracy. In fact, it outperformed the standard method based on tumor diameter, known as Response Evaluation Criteria in Solid Tumors 1.1 (RECIST 1.1), which is commonly used in clinical trials to assess treatment efficacy.
“The field of radiology and imaging in general has never been more exciting with this artificial intelligence revolution,” says Dr. Schwartz. “We’ve always looked at advances in terms of new machines, new tracers, and things like this. But this gives us an opportunity to optimize the information that we have from all of our imaging modalities to speed diagnosis, to become more accurate and precise, and give patients more effective treatments.”
Meeri Kim, Columbia University