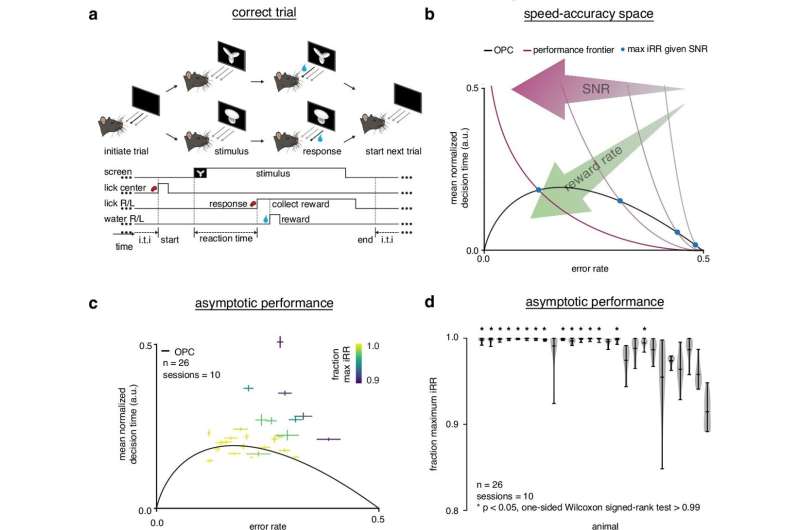
Scientists have provided evidence for the cognitive control of learning in rats, showing they can estimate the long-term value of learning and adapt their decision-making strategy to take advantage of learning opportunities.
The findings suggest that by taking longer over a decision, rats may sacrifice immediate rewards to increase their learning outcomes and achieve greater rewards over the entire course of a task. The results are published today in eLife.
An established principle of behavioral neuroscience is the speed-accuracy trade-off, which is seen across many species, from rodents to primates. The principle describes the relationship between an individual’s willingness to respond slowly and make fewer errors compared to their willingness to respond quickly and risk making more errors.
“Many studies in this area have focused on the speed-accuracy trade-off, without taking learning outcomes into account,” says lead author Javier Masís, who at the time was a Ph.D. student at the Department of Molecular and Cellular Biology, and the Center for Brain Science, Harvard University, US, and is now a Presidential Postdoctoral Research Fellow at the Princeton Neuroscience Institute at Princeton University, U.S. “We aimed to investigate the difficult intertemporal choice problem that exists when you have the possibility to improve your behavior through learning.”
For their study, Masís and colleagues sought to first establish whether rats were able to solve the speed-accuracy trade-off. The team set up an experiment where rats, upon seeing one of two visual objects that could vary in their size and rotation, decided whether the visual object was the one that corresponded to a left response, or a right response, and licked the corresponding touch-sensitive port once they had decided. If the rats licked the correct port, they were rewarded with water, and if they licked the wrong port, they were given a timeout.
The team investigated the relationship between error rate (ER) and reaction time (RT) during these trials, using the Drift-Diffusion Model (DDM)—a standard decision-making model in psychology and neuroscience in which the decision maker accumulates evidence through time until the level of evidence for one alternative reaches a threshold.
The subject’s threshold level controls the speed-accuracy trade-off. Using a low-threshold yields fast but error-prone responses, whereas a high-threshold yields slow, but accurate responses. For every difficulty level, however, there is a best threshold to set that optimally balances speed and accuracy, allowing the decision maker to maximize their instantaneous reward rate (iRR). Across difficulties, this behavior can be summarized through a relationship between ER and RT called the optimal performance curve (OPC). After learning the task fully, over half of the trained rats reached the OPC, demonstrating that well-trained rats solve the speed-accuracy trade-off.
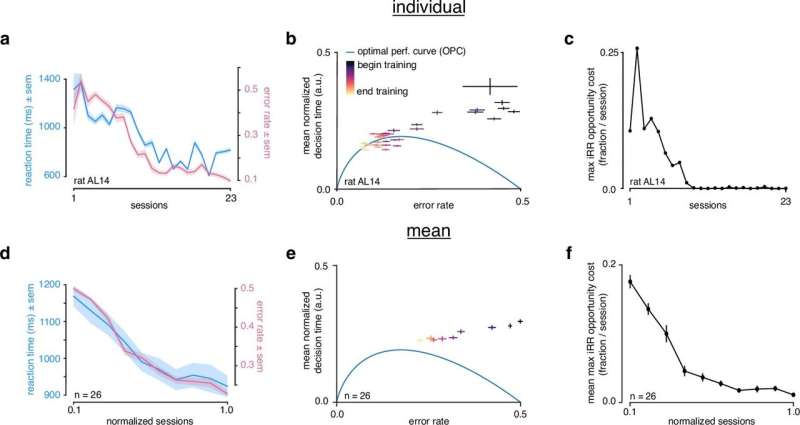
At the start of training, though, all rats gave up over 20% of their iRR, whereas towards the end, most rats near optimally maximized iRR. This prompted the question: If rats maximize instantaneous rewards by the end of learning, what governs their strategy at the beginning of learning?
To answer this, the team adapted the DDM as a recurrent neural network (RNN) that could learn over time and developed the Learning Drift-Diffusion Model (LDDM), enabling them to investigate how long-term perceptual learning across many trials is influenced by the choice of decision time in individual trials.
The model was designed with simplicity in mind, to highlight key qualitative trade-offs between learning speed and decision strategy. The analyses from this model suggested that rats adopt a “non-greedy” strategy that trades initial rewards to prioritize learning and therefore maximize total reward over the course of the task. They also demonstrated that longer initial reaction times lead to faster learning and higher reward, both in an experimental and simulated environment.
The authors call for further studies to consolidate these findings. The current study is limited by the use of the DDM to estimate improved learning. The DDM, and therefore LDDM, is a simple model that is a powerful theoretical tool for understanding specific types of simple choice behavior that can be studied in the lab, but it is not capable of quantitatively describing more naturalistic decision-making behavior. Furthermore, the study focuses on one visual perceptual task; the authors therefore encourage further work with other learnable tasks across difficulties, sensory modalities and organisms.
“Our results provide a new view of the speed-accuracy trade-off by showing that perceptual decision-making behavior is strongly shaped by the stringent requirement to learn quickly,” claims senior author Andrew Saxe, previously a postdoctoral research associate at the Department of Experimental Psychology, University of Oxford, UK, and now Sir Henry Dale Fellow and Associate Professor at the Gatsby Computational Unit and Sainsbury Wellcome Center, University College London, UK.
“A key principle that our study propounds,” explains Javier Masís, “is that natural agents take into account the fact that they can improve through learning, and that they can and do shape the rate of that improvement through their choices. Not only is the world we live in non-stationary; we are also non-stationary, and we take that into account as we move around the world making choices.”
“You don’t learn the piano by futzing around the keys occasionally,” adds Saxe. “You decide to practice, and you practice at the expense of other more immediately rewarding activities because you know you’ll improve and it’ll probably be worth it in the end.”
eLife