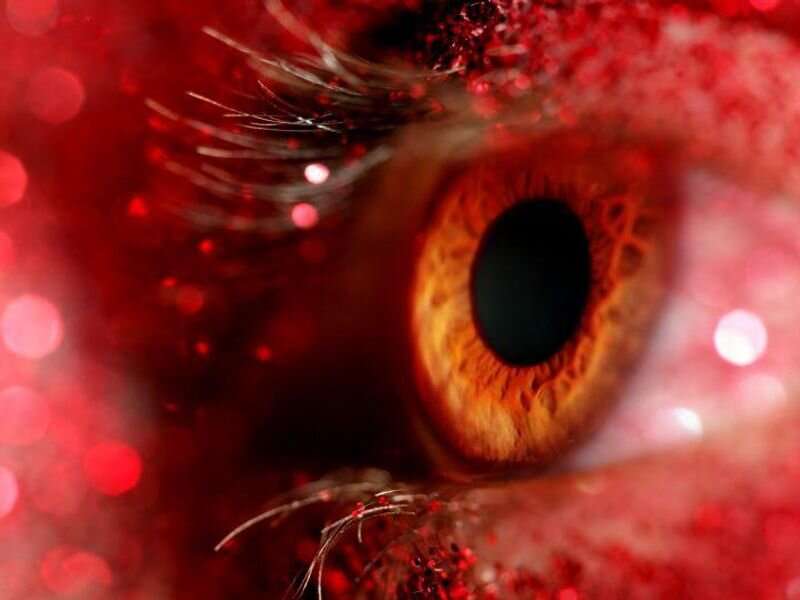
Artificial intelligence (AI) algorithms trained on fundus photographs infer the self-reported race (SRR) of infants, regardless of whether the images contain color, according to a study published online May 4 in JAMA Ophthalmology.
Aaron S. Coyner, Ph.D., from Oregon Health & Science University in Portland, and colleagues examined whether converting color fundus photographs to retinal vessel maps (RVMs) of infants screened for retinopathy of prematurity removes the risk for racial bias. The major arteries and veins in retinal fundus images (RFIs) were segmented into grayscale RVMs using a convolutional neural network (CNNs), u-net, which provides precise segmentation for biomedical images. A total of 4,095 RFIs were obtained from 245 neonates with parent-reported Black or White race (38.4 and 61.6 percent, respectively).
The researchers found that CNNs inferred SRR almost perfectly from RFIs (image-level area under the precision recall curve [AUC-PR], 0.999; infant-level AUC-PR, 1.000). Compared with color RFIs, raw RVMs were nearly as informative (image-level AUC-PR, 0.938; infant-level AUC-PR, 0.995). Regardless of whether images contained color, vessel segmentation brightness differences were nullified, or vessel segmentation widths were uniform, CNNs were able to learn whether RFIs or RVMs were from Black or White infants.
“Results of this diagnostic study suggest that it can be very challenging to remove information relevant to SRR from fundus photographs,” the authors write. “As a result, AI algorithms trained on fundus photographs have the potential for biased performance in practice, even if based on biomarkers rather than raw images.”
Several authors disclosed financial ties to the pharmaceutical and medical technology industries.
Elana Gotkine