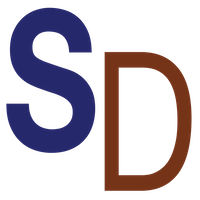
Researchers who had been using Fitbit data to help predict surgical outcomes have a new method to more accurately gauge how patients may recover from spine surgery.
Using machine learning techniques developed at the AI for Health Institute at Washington University in St. Louis, Chenyang Lu, the Fullgraf Professor in the university’s McKelvey School of Engineering, collaborated with Jacob Greenberg, MD, assistant professor of neurosurgery at the School of Medicine, to develop a way to predict recovery more accurately from lumbar spine surgery.
The results published this month in the journal Proceedings of the ACM on Interactive, Mobile, Wearable and Ubiquitous Technologies, show that their model outperforms previous models to predict spine surgery outcomes. This is important because in lower back surgery and many other types of orthopedic operations, the outcomes vary widely depending on the patient’s structural disease but also varying physical and mental health characteristics across patients.
Surgical recovery is influenced by both preoperative physical and mental health. Some people may have catastrophizing, or excessive worry, in the face of pain that can make pain and recovery worse. Others may suffer from physiological problems that cause worse pain. If physicians can get a heads-up on the various pitfalls for each patient, that will allow for better individualized treatment plans.
“By predicting the outcomes before the surgery, we can help establish some expectations and help with early interventions and identify high risk factors,” said Ziqi Xu, a PhD student in Lu’s lab and first author on the paper.
Previous work in predicting surgery outcomes typically used patient questionnaires given once or twice in clinics that capture only one static slice of time.
“It failed to capture the long-term dynamics of physical and psychological patterns of the patients,” Xu said. Prior work training machine learning algorithms focus on just one aspect of surgery outcome “but ignore the inherent multidimensional nature of surgery recovery,” she added.
Researchers have used mobile health data from Fitbit devices to monitor and measure recovery and compare activity levels over time but this research has shown that activity data, plus longitudinal assessment data, is more accurate in predicting how the patient will do after surgery, Greenberg said.
The current work offers a “proof of principle” showing, with the multimodal machine learning, doctors can see a much more accurate “big picture” of all the interrelated factors that affect recovery. Proceeding this work, the team first laid out the statistical methods and protocol to ensure they were feeding the AI the right balanced diet of data.
Prior to the current publication, the team published an initial proof of principle in Neurosurgery showing that patient-reported and objective wearable measurements improve predictions of early recovery compared to traditional patient assessments. In addition to Greenberg and Xu, Madelynn Frumkin, a PhD psychological and brain sciences student in Thomas Rodebaugh’s laboratory in Arts & Sciences, was co-first author on that work. Wilson “Zack” Ray, MD, the Henry G. and Edith R. Schwartz Professor of neurosurgery in the School of Medicine, was co-senior author, along with Rodebaugh and Lu. Rodebaugh is now at the University of North Carolina at Chapel Hill.
In that research, they show that Fitbit data can be correlated with multiple surveys that assess a person’s social and emotional state. They collected that data via “ecological momentary assessments” (EMAs) that employ smart phones to give patients frequent prompts to assess mood, pain levels and behavior multiple times throughout day.
“We combine wearables, EMA -and clinical records to capture a broad range of information about the patients, from physical activities to subjective reports of pain and mental health, and to clinical characteristics,” Lu said.
Greenberg added that state-of-the-art statistical tools that Rodebaugh and Frumkin have helped advance, such as “Dynamic Structural Equation Modeling,” were key in analyzing the complex, longitudinal EMA data.
For the most recent study they then took all those factors and developed a new machine learning technique of “Multi-Modal Multi-Task Learning (M3TL)” to effectively combine these different types of data to predict multiple recovery outcomes.
In this approach, the AI learns to weigh the relatedness among the outcomes while capturing their differences from the multimodal data, Lu adds.
This method takes shared information on interrelated tasks of predicting different outcomes and then leverages the shared information to help the model understand how to make an accurate prediction, according to Xu.
It all comes together in the final package producing a predicted change for each patient’s post-operative pain interference and physical function score.
Greenberg says the study is ongoing as they continue to fine tune their models so they can take these more detailed assessments, predict outcomes and, most notably, “understand what types of factors can potentially be modified to improve longer term outcomes.”
This study was funded by grants from AO Spine North America, the Cervical Spine Research Society, the Scoliosis Research Society, the Foundation for Barnes-Jewish Hospital, Washington University/BJC Healthcare Big Ideas Competition, the Fullgraf Foundation, and the National Institute of Mental Health (1F31MH124291-01A).