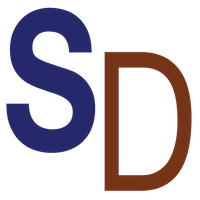
Large language models may pass medical exams with flying colors but using them for diagnoses would currently be grossly negligent. Medical chatbots make hasty diagnoses, do not adhere to guidelines, and would put patients’ lives at risk. This is the conclusion reached by a team from the Technical University of Munich (TUM). For the first time, the team has systematically investigated whether this form of artificial intelligence (AI) would be suitable for everyday clinical practice. Despite the current shortcomings, the researchers see potential in the technology. They have published a method that can be used to test the reliability of future medical chatbots.
Large language models are computer programs trained with massive amounts of text. Specially trained variants of the technology behind ChatGPT now even solve final exams from medical studies almost flawlessly. But would such an AI be able to take over the tasks of doctors in an emergency room? Could it order the appropriate tests, make the right diagnosis, and create a treatment plan based on the patient’s symptoms?
An interdisciplinary team led by Daniel Rückert, Professor of Artificial Intelligence in Healthcare and Medicine at TUM, addressed this question in the journal Nature Medicine. For the first time, doctors and AI experts systematically investigated how successful different variants of the open-source large language model Llama 2 are in making diagnoses
Reenacting the path from emergency room to treatment
To test the capabilities of these complex algorithms, the researchers used anonymized patient data from a clinic in the USA. They selected 2400 cases from a larger data set. All patients had come to the emergency room with abdominal pain. Each case description ended with one of four diagnoses and a treatment plan. All the data recorded for the diagnosis was available for the cases — from the medical history and blood values to the imaging data.
“We prepared the data in such a way that the algorithms were able to simulate the real procedures and decision-making processes in the hospital,” explains Friederike Jungmann, assistant physician in the radiology department at TUM’s Klinikum rechts der Isar and lead author of the study together with computer scientist Paul Hager. “The program only had the information that the real doctors had. For example, it had to decide for itself whether to order a blood count and then use this information to make the next decision — until it finally created a diagnosis and a treatment plan.”
The team found that none of the large language models consistently requested all the necessary examinations. In fact, the programs’ diagnoses became less accurate the more information they had about the case. They often did not follow treatment guidelines, sometimes ordering examinations that would have had serious health consequences for real patients.
Direct comparison with doctors
In the second part of the study, the researchers compared AI diagnoses for a subset of the data with diagnoses from four doctors. While the latter were correct in 89 percent of the diagnoses, the best large language model achieved just 73 percent. Each model recognized some diseases better than others. In one extreme case, a model correctly diagnosed gallbladder inflammation in only 13 percent of cases.
Another problem that disqualifies the programs for everyday use is a lack of robustness: the diagnosis made by a large language model depended, among other things, on the order in which it received the information. Linguistic subtleties also influenced the result — for example, whether the program was asked for a ‘Main Diagnosis,’ a ‘Primary Diagnosis,’ or a ‘Final Diagnosis.’ In everyday clinical practice, these terms are usually interchangeable.
ChatGPT not tested
The team explicitly did not test the commercial large language models from OpenAI (ChatGPT) and Google for two main reasons. Firstly, the provider of the hospital data has prohibited the data from being processed with these models for data protection reasons. Secondly, experts strongly advise that only open-source software should be used for applications in the healthcare sector. “Only with open-source models do hospitals have sufficient control and knowledge to ensure patient safety. When we test models, it is essential to know what data was used to train them. Otherwise, we might test them with the exact same questions and answers they were trained on. Companies of course keep their training data very secret, making fair evaluations hard,” says Paul Hager. “Furthermore, basing key medical infrastructure on external services which update and change models as they wish is dangerous. In the worst-case scenario, a service on which hundreds of clinics depend could be shut down because it is not profitable.”
Rapid progress
Developments in this technology are advancing rapidly. “It is quite possible that in the foreseeable future a large language model will be better suited to arriving at a diagnosis from medical history and test results,” says Prof. Daniel Rückert. “We have therefore released our test environment for all research groups that want to test large language models in a clinical context.” Rückert sees potential in the technology: “In the future, large language models could become important tools for doctors, for example for discussing a case. However, we must always be aware of the limitations and peculiarities of this technology and consider these when creating applications,’ says the medical AI expert.”