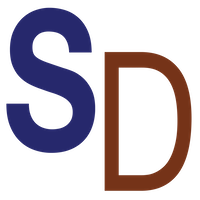
The human genetic code is fully mapped out, providing scientists with a blueprint of the DNA to identify genomic regions and their variations responsible for diseases. Traditional statistical tools effectively pinpoint these genetic “needles in the haystack,” yet they face challenges in understanding how many genes contribute to diseases, as seen in diabetes or schizophrenia. A new study from the Institute of Science and Technology Austria (ISTA), published in PNAS, tackles this problem.
Many statistical models and algorithms used by scientists can be imagined as a “black box.” These models are powerful tools that give accurate predictions, but their internal workings are not easily interpretable or understood. In an era dominated by deep learning, where an ever-increasing amount of data can be processed, Natália Ružičková, a physicist and PhD student at the Institute of Science and Technology Austria (ISTA), chose to take a step back. At least in the context of genomic data analysis.
Together with Michal Hledík, a recent ISTA graduate, and Professor Gašper Tkačik, Ružičková now proposed a model that might help to analyze “polygenic diseases,” where many regions in the genome contribute to a malfunction. Also, the model serves to understand why the identified genomic regions contribute to these diseases. They do so by combining state-of-the-art genome analysis with fundamental biology insights. The results are published in PNAS.
Decoding the human genome
In 1990, the Human Genome Project was launched to fully decode the human DNA — the genetic blueprint that defines humans. Fast-forward to 2003 when the project was completed, it paved the way for numerous breakthroughs in science, medicine, and technology. By deciphering the human genetic code, scientists were hopeful to learn more about diseases linked to specific mutations and variations in this genetic script. Given that the human genome comprises approximately 20,000 genes and even more base pairs — the letters of the blueprint — large statistical power became essential. This led to the development of so-called “genome-wide association studies” (GWAS).
GWAS approach the issue by identifying genetic variants potentially linked to organismal traits such as height. Importantly, they also include the propensity for various diseases. For this, the underlying statistical principle is quite straightforward: participants are divided into two groups — healthy and sick individuals. Their DNA is then analyzed to detect variations — changes in their genome — that are more prominent in those affected by the disease.
An interplay of genes
When genome-wide association studies emerged, scientists expected to find just a few mutations in known genes linked to a disease that would explain the difference between healthy and sick individuals. The truth, however, is much more complicated. “Sometimes, there are hundreds or thousands of mutations linked to a specific disease,” says Ružičková. “It was a surprising revelation and conflicted with the understanding of biology we had.”
Individually, each mutation has a minimal impact or contribution to the risk of developing a disease. However, collectively, they can explain better, but not fully, why some individuals develop the disease. Such diseases are referred to as “polygenic.” For example, type 2 diabetes is polygenic, because it cannot be attributed to a single gene; instead, it involves hundreds of mutations. Some of these mutations affect insulin production, insulin action, or glucose metabolism, while the majority are located in genomic regions not previously linked to diabetes or with unknown biological functions.
The omnigenic model
In 2017, Evan A. Boyle and colleagues from Stanford University proposed a new conceptual framework called the “omnigenic model.” They proposed an explanation for why so many genes contribute to diseases: cells possess regulatory networks that link genes with diverse functions.
“Since genes are interconnected, a mutation in one gene can impact other ones, as the mutational effect spreads through the regulatory network,” Ružičková explains. Due to these networks, many genes in the regulatory system end up contributing to a disease. However, until now, this model has not been formulated mathematically and has remained a conceptual hypothesis that was difficult to test. In their latest paper, Ružičková and her colleagues introduce a new mathematical formalization based on the omnigenic model named the “quantitative omnigenic model” (QOM).
Combining statistics and biology
To demonstrate the potential of the new model, they needed to apply the framework to a well-characterized biological system. They chose the common lab yeast model Saccharomyces cerevisiae, better known as the brewer’s yeast or the baker’s yeast. It is a single-cell eukaryote, meaning its cell structure is similar to that of complex organisms such as humans. “In yeast, we have a fairly good understanding of how regulatory networks that interconnect genes are structured,” Ružičková says.
Using their model, the scientists predicted gene expression levels — the intensity of gene activity, indicating how much information from the DNA is actively utilized — and how mutations spread through the yeast’s regulatory network. The predictions were highly efficient: The model not only identified the relevant genes but could also clearly pinpoint which mutation most likely contributed to a specific outcome.
The puzzle pieces of polygenic diseases
The scientists’ goal was not to outdo the standard GWAS in prediction performance, but rather to go in a different direction by making the model interpretable. Whereas a standard GWAS model works as a “black box,” offering a statistical account of how frequently a particular mutation is linked to a disease, the new model also provides a chain-of-events causal mechanism how that mutation may lead to a disease.
In medicine, understanding the biological context and such causal pathways has huge implications for finding new therapeutic options. Although the model is currently far from any medical application, it shows potential, especially for learning more about polygenic diseases. “If you have enough knowledge about the regulatory networks, you could build similar models for other organisms as well. We looked at the gene expression in yeast, which is just the first step and proof of principle. Now that we understand what is possible, one can start thinking about applications to human genetics,” says Ružičková.