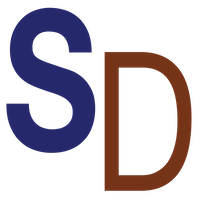
State-of-the-art artificial intelligence systems known as large language models (LLMs) are poor medical coders, according to researchers at the Icahn School of Medicine at Mount Sinai. Their study, published in the April 19 online issue of NEJM AI, emphasizes the necessity for refinement and validation of these technologies before considering clinical implementation.
The study extracted a list of more than 27,000 unique diagnosis and procedure codes from 12 months of routine care in the Mount Sinai Health System, while excluding identifiable patient data. Using the description for each code, the researchers prompted models from OpenAI, Google, and Meta to output the most accurate medical codes. The generated codes were compared with the original codes and errors were analyzed for any patterns.
The investigators reported that all of the studied large language models, including GPT-4, GPT-3.5, Gemini-pro, and Llama-2-70b, showed limited accuracy (below 50 percent) in reproducing the original medical codes, highlighting a significant gap in their usefulness for medical coding. GPT-4 demonstrated the best performance, with the highest exact match rates for ICD-9-CM (45.9 percent), ICD-10-CM (33.9 percent), and CPT codes (49.8 percent).
GPT-4 also produced the highest proportion of incorrectly generated codes that still conveyed the correct meaning. For example, when given the ICD-9-CM description “nodular prostate without urinary obstruction,” GPT-4 generated a code for “nodular prostate,” showcasing its comparatively nuanced understanding of medical terminology. However, even considering these technically correct codes, an unacceptably large number of errors remained.
The next best-performing model, GPT-3.5, had the greatest tendency toward being vague. It had the highest proportion of incorrectly generated codes that were accurate but more general in nature compared to the precise codes. In this case, when provided with the ICD-9-CM description “unspecified adverse effect of anesthesia,” GPT-3.5 generated a code for “other specified adverse effects, not elsewhere classified.”
“Our findings underscore the critical need for rigorous evaluation and refinement before deploying AI technologies in sensitive operational areas like medical coding,” says study corresponding author Ali Soroush, MD, MS, Assistant Professor of Data-Driven and Digital Medicine (D3M), and Medicine (Gastroenterology), at Icahn Mount Sinai. “While AI holds great potential, it must be approached with caution and ongoing development to ensure its reliability and efficacy in health care.”
One potential application for these models in the health care industry, say the investigators, is automating the assignment of medical codes for reimbursement and research purposes based on clinical text.
“Previous studies indicate that newer large language models struggle with numerical tasks. However, the extent of their accuracy in assigning medical codes from clinical text had not been thoroughly investigated across different models,” says co-senior author Eyal Klang, MD, Director of the D3M’s Generative AI Research Program. “Therefore, our aim was to assess whether these models could effectively perform the fundamental task of matching a medical code to its corresponding official text description.”
The study authors proposed that integrating LLMs with expert knowledge could automate medical code extraction, potentially enhancing billing accuracy and reducing administrative costs in health care.
“This study sheds light on the current capabilities and challenges of AI in health care, emphasizing the need for careful consideration and additional refinement prior to widespread adoption,” says co-senior author Girish Nadkarni, MD, MPH, Irene and Dr. Arthur M. Fishberg Professor of Medicine at Icahn Mount Sinai, Director of The Charles Bronfman Institute of Personalized Medicine, and System Chief of D3M.
The researchers caution that the study’s artificial task may not fully represent real-world scenarios where LLM performance could be worse.
Next, the research team plans to develop tailored LLM tools for accurate medical data extraction and billing code assignment, aiming to improve quality and efficiency in health care operations.
The study is titled “Generative Large Language Models are Poor Medical Coders: A Benchmarking Analysis of Medical Code Querying.”
The remaining authors on the paper, all with Icahn Mount Sinai except where indicated, are: Benjamin S. Glicksberg, PhD; Eyal Zimlichman, MD (Sheba Medical Center and Tel Aviv University, Israel); Yiftach Barash, (Tel Aviv University and Sheba Medical Center, Israel); Robert Freeman, RN, MSN, NE-BC; and Alexander W. Charney, MD, PhD.
This research was supported by the AGA Research Foundation’s 2023 AGA-Amgen Fellowship to-Faculty Transition Award AGA2023-32-06 and an NIH UL1TR004419 award.
The researchers affirm that the study was conducted without the use of any Protected Health Information (“PHI”).